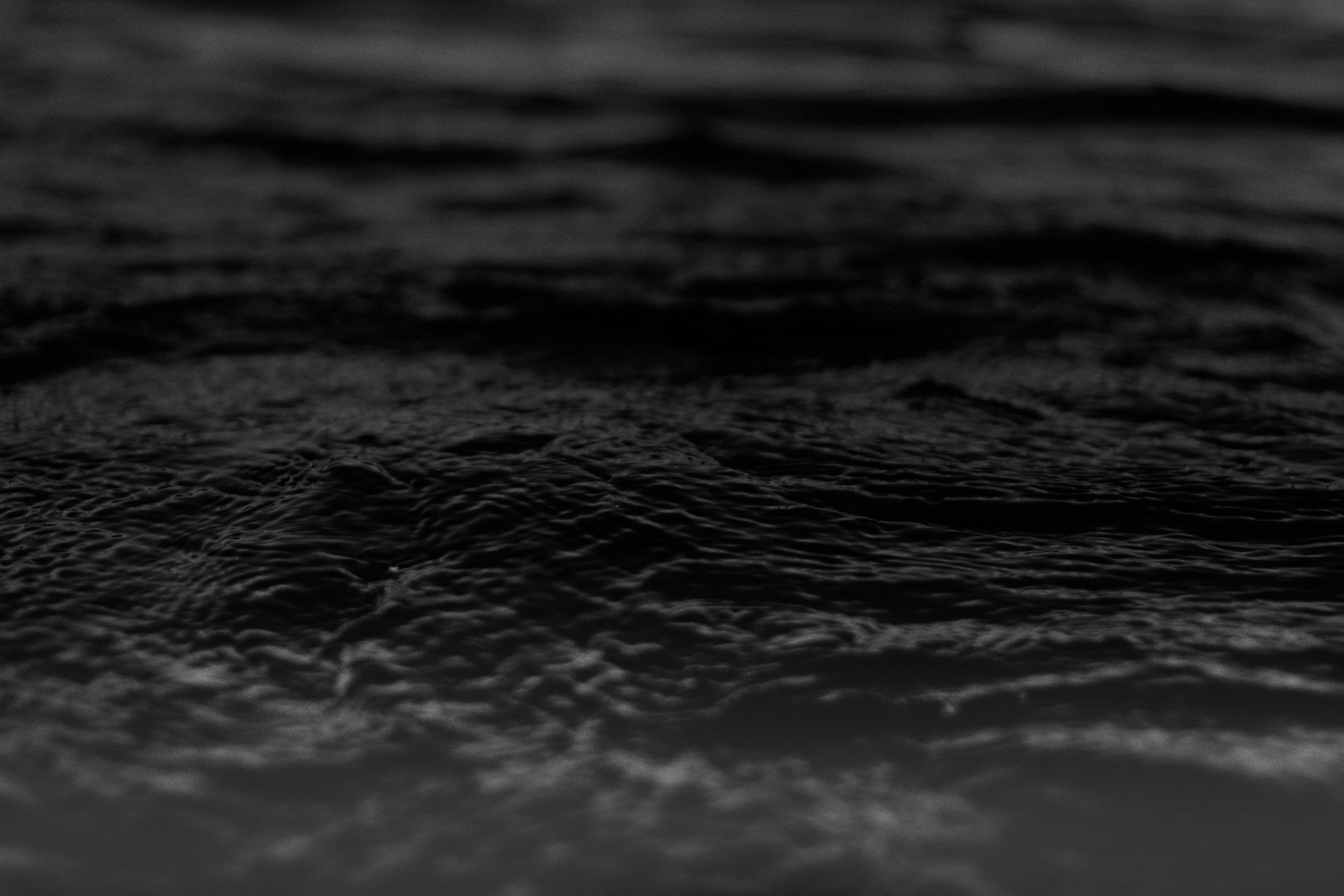
There is no passion to be found playing small
--- NELSON MANDELA
Research Themes
Humans (and many non-human animals) are apt at learning cumulatively and exploiting experiences prospectively to generate goal-directed behaviors with flexibility and creativity. Understanding the computational processes in brains that give rise to these cognitive behaviors and endowing artificial agents (robots) with such brain-like mechanisms to enable them act cognitively like (and alongside) natural agents, form the long-term goals of my research. I explore these core problems by blending studies from cognitive neurosciences and experiments from developmental psychology into cognitive robotics (see Figure 1) to investigate the organization of action, learning and memory in cumulatively developing systems (animals and robots). Imbibing emerging trends from neurosciences I endeavor to design brain-guided cognitive architectures, validate them by reenacting on humanoid robots, experiments from infant and animal cognition, and finally port the ensuing developments into application domains. The research is of significance in understanding the brain-behavior relationship better and in designing cognitive artifacts that assist us in environments we inhabit and create, ultimately enhancing the quality of life.
Looking at cognition from a holistic perspective and cognitive agents as integrated systems has led me contribute to many subdomains over the three years of my doctoral study, in particular following ones:
Semantic Memory / Object Concepts
​How are object concepts represented in our brain? Mounting evidence from functional imaging and connectomics suggests a) semantic knowledge is grounded in a distributed fashion in modality/property-specific cortical networks that directly support perception and action and that were active during learning1; and b) brain exhibits small world topology to support segregated/specialized and distributed/integrated information processing2. With my supervisor, I worked on a model imbibing these key principles that allow functional segregation of property features (e.g. color, shape, size, weight etc.) and their global integration at multi-modal hubs into object concepts. Through a network dynamics for cross-modal, top-down and bottom-up communication, the proposed small-world of self-organizing maps facilitates learning of object concepts and both real perception and imagination of leant as well as novel objects.
Development of Word Learning
How do infants acquire vocabulary progressively despite substantial referential uncertainty in what they hear and see? And if this learning happens through statistical co-occurrence tracking as suggested in the literature (19), what neural mechanisms underlie these forms of cross-situational word learning? In this context, I with my colleagues propose a neurally grounded process account (WOLVES) of early vocabulary acquisition that integrates Word-Object Learning with Visual Exploration in Space across multiple timescales (20). Grounded in a dynamical systems framework, the model addresses how top-down & bottom-up attention, working memory and long-term memory traces influences word learning and moment-to-moment looking behaviours; and how these systems co-evolve over development. Focusing on cross-situational word learning, WOLVES model successfully captures the visual dynamics and learning behaviour of infants (see Figure 2) as reported in (19) such as the number of words learned by strong and weak learners, looking times to targets vs distractors, fixation counts and duration of fixations etc.
Episodic Memory
What are the neural representations of sensory-motor experiences, how are they encoded, recalled and reused? Emerging trends in neuroscience indicate that episodic memory circuit involves higher-cortical areas to process sensorimotor information, interfacing para-hippocampal regions/hubs to communicate with the hippocampus and the hippocampus to integrate, encode and recall information within an experience3. Additionally, converging evidence suggests that a network of cortical hubs (including para-hippocampal areas and hippocampus) known as Default Mode Network4 (DMN) is shared while recalling the past and prospective simulation of the future, goal-directed planning and creative thinking. With my supervisor, I worked on a model of episodic memory system that emulates the brain-like memory architecture and also mimics the DMN by engaging a network of hubs (representing actions on various objects involved, the ensuing consequences, internal state of body, and rewards received) during encoding, recall and goal-directed reuse of experiences.
Reasoning & Creativity
​How do cognitive agents exploit their past experiences in the context of present to realize goals and reason into future to solve non-trivial problems creatively? A classic experiment from psychology investigating novel behavior was on Betty5, that when faced with a non-trivial problem to pull out her dinner basket trapped in a vertical tube, recalled her year-old experience of bending flexible pipe cleaners to shape a hook out of a wire lying nearby and thereby was able to pull out the basket. Mimicking the Betty task on iCub, I with my supervisor designed two experiments investigating the reasoning and creativity capabilities of the proposed memory architecture.
Causal Learning & Inference​
How over cumulating experiences, causal relations between goals, actions and the objects in the environment are both abstracted and then exploited in novel contexts? Several experiments from psychology like the crow and Pitcher6 task (from Aesop's Fables) show that corvids, apes as well as children are able to learn and infer causal-effect relations. Reenacting this Aesop's fable task on iCub humanoid in the context of open-ended learning-prediction-abstraction loop, I and my supervisor investigated the problem by employing the memory architecture and proposing four task-agnostic learning rules (elimination, growth, uncertainty, status-quo) that correlate predictions from remembered past experiences in the context of present to abstract underlying causal relations. The ensuing robot behaviors were found strikingly similar to children. Results further demonstrate how by cumulatively interacting with few objects, the predictions of the robot in case of novel objects converges close to the physical law i.e. the Archimedes principle.
Motor Control & Cognition
How does the brain coordinate action in redundant bodies as well as anticipate and understand actions of self and others? Emerging trends in motor neurosciences provide converging evidence that cortical networks in predominantly motor areas are also activated in imagination and observation of actions without causing any overt movement. Revisiting older ideas from motor control like Equilibrium Point Hypothesis and synergy formation in light of these emerging trends, I worked on a framework proposing that actions are consequences of a simulation process that animates a plastic, expandable, configurable internal model of the body (namely the body schema), under the force-fields of intended goals/constraints. The framework provides a unified computational basis for actions with and without movements through emergence of muscle-less motor synergies thus facilitating a seamless continuum between motor control and motor imagery.
Spatial Planning & Cooperation
How can multiple agents in spatially unstructured and temporally evolving shared environments plan their actions and cooperate to realize common goals? In a real-world industrial setting where multiple robots are jointly operating in a shared workspace to perform a joint assembly, I worked on the design of a biomimetic architecture that operates through coupled interactions between the robots’ body model (schema) and an internal model of its peripersonal space. Both the internal models are jointly learnt through sensorimotor exploration and then based on a reward field dynamics are engaged in a range of inferences related to feasibility and consequence of potential actions of one self and other to both jointly or individually complete as many assemblies as possible and maximize their individual rewards.
-
Bhat, A. A.., J Spencer, L Samuelson (2020). Cross-situational word learning models: A review, in preparation
-
Bhat, A. A., J Spencer, L Samuelson (2020). WOLVES: A neuro-computational model of children’s response to novelty and word learning, submitted
-
Bhat, A. A., J Spencer, L Samuelson (2020). Word-Object Learning via Visual Exploration in Space (WOLVES): A Neural Process Account of Cross-Situational Word Learning. in review Psychological Review []
-
Bhat, A. A., Mohan V. (2020). Bending it Like Betty: Memory-based Cognition in natural and artificial agents, in prep
-
Bhat, A. A., Mohan V. (2020). Instructable cognitive machines for multi-task learning, in prep Künstliche Intelligenz
-
Bhat, A. A., Mohan V. (2020). Creative Problem-Solving in Robots using Constructive Episodic Simulations. In review, 29th IEEE International Conference on Robot and Human Interactive Communication, Naples.
-
Bhat, A. A., Spencer, J. P., & Samuelson, L. K. (2020). Effect of Metric Variation in Object Shapes and Colours on Cross-Situational Word Learning in Adults. OSF Pre-registry. Retrieved from https://osf.io/rbg7m
-
Bhat, A. A., Spencer, J. P., & Samuelson, L. K. (2020). Moving beyond Associative Learning and Hypothesis Testing: How Stimulus Exposure Times and Looking Behaviours Conspire in Cross-Situational Word Learning. OSF Pre-registry. Retrieved from https://osf.io/htd7p
-
Bhat, A. A., Mohan V. (2020). Causal Learning by a Robot with Semantic-Episodic Memory in an Aesop's Fable Experiment, International Conference on Learning and Representation, (ICLR), Addis Ababa arXiv:2003.00274 https://arxiv.org/abs/2003.00274
-
Mohan V., Morasso P., Bhat, A. A. (2019). The complementarity of ‘Muscleless’ motor synergies with motor control strategies in humans and robots: Reply to Comments Physics of Life Reviews, ISSN 1571-0645, https://doi.org/10.1016/j.plrev.2019.11.001.
-
Mohan V., Bhat, A. A., Morasso P. (2019). Muscle-less motor synergies and actions without movements. From motor neuroscience to embodied cognition. Physics of Life Reviews. https://doi.org/10.1016/j.plrev.2018.04.005
-
Mohan V., Bhat, A. A. (2019). Why human Tools, Scripts and Skills are the way they are? Insights from teaching motor skills to humanoid robots. In review, Cognitive Science.
-
Bhat, A. A. Mohan V. (2018). Goal directed reasoning and cooperation in robots in shared workspaces: An internal simulation based neural framework, Cognitive Computation https://doi.org/10.1007/s12559-018-9553-1
-
Mohan, V., & Bhat, A. A. (2018). Joint Goal Human-Robot collaboration-From Remembering to Inferring. 8th Annual International Conference on Biologically Inspired Cognitive Architectures BICA 2017, published in Procedia Computer Science, 123, 579-584. [Full paper] https://doi.org/10.1016/j.procs.2018.01.089
-
Bhat, A. A., Spencer, J. P, & Samuelson, L. K. (2018). A dynamic neural field model of memory, attention and cross-situational word learning. CogSci 2018: Madison, The 40th Annual Meeting of the Cognitive Science Society. [Full Paper] http://mindmodeling.org/cogsci2018/papers/0048/index.html
-
Bhat, A. A., Mohan V., Akkaladevi S. C., Eitzinger C., Sandini G., Morasso P. (2016). Towards a learnt neural Body schema for dexterous coordination of action in Humanoid and Industrial robots. Autonomous Robots 41: 945 http://link.springer.com/article/10.1007/s10514-016-9563-3
-
Bhat, A. A., Mohan V., Sandini G., Morasso P. (2016). Humanoid robot infers Archimedes’ principle: From cumulative exploration to abstraction of underlying physical relations and object affordances. Journal of the Royal Society Interface 13: 20160310 http://rsif.royalsocietypublishing.org/content/13/120/20160310
-
Bhat, A. A., Mohan V., (2015). How iCub Learns to Imitate Use of a Tool Quickly by Recycling the Past Knowledge Learnt During Drawing. In Biomimetic and Biohybrid Systems, pp. 339-347. Springer International Publishing. http://dx.doi.org/10.1007/978-3-319-22979-9_33
-
Bhat, A. A., Mohan V., Rea F., Morasso P., Sandini G. (2014). “Connecting Experiences”: Towards a Biologically Inspired Episodic Memory for Developmental Robots". IEEE International Conf. on Development and Learning (ICDL-EPIROB 2014), Genoa, Italy. [Full paper] https://ieeexplore.ieee.org/document/6983007
-
Mohan V., Bhat, A. A., Morasso P., Sandini G. (2014). From Object-Action to Property-Action: Learning causally dominant properties through cumulative explorative interactions, Biologically Inspired Cognitive Architectures 10, p42-50. http://dx.doi.org/10.1016/j.bica.2014.11.006
-
Bhat, A. A., Mehta A., (2012). Dynamics of Competitive Learning: The role of Updates and Memory. Physical Review E 85, 011134. http://dx.doi.org/10.1103/PhysRevE.85.011134
-
Bhat, A. A., Mahajan G., Mehta A., (2011). Learning with a network of Competing Synapses, PLoS ONE 6(9): e25048. http://dx.doi.org/10.1371/journal.pone.0025048